What is AI Lead Scoring & How to Use it (2024)
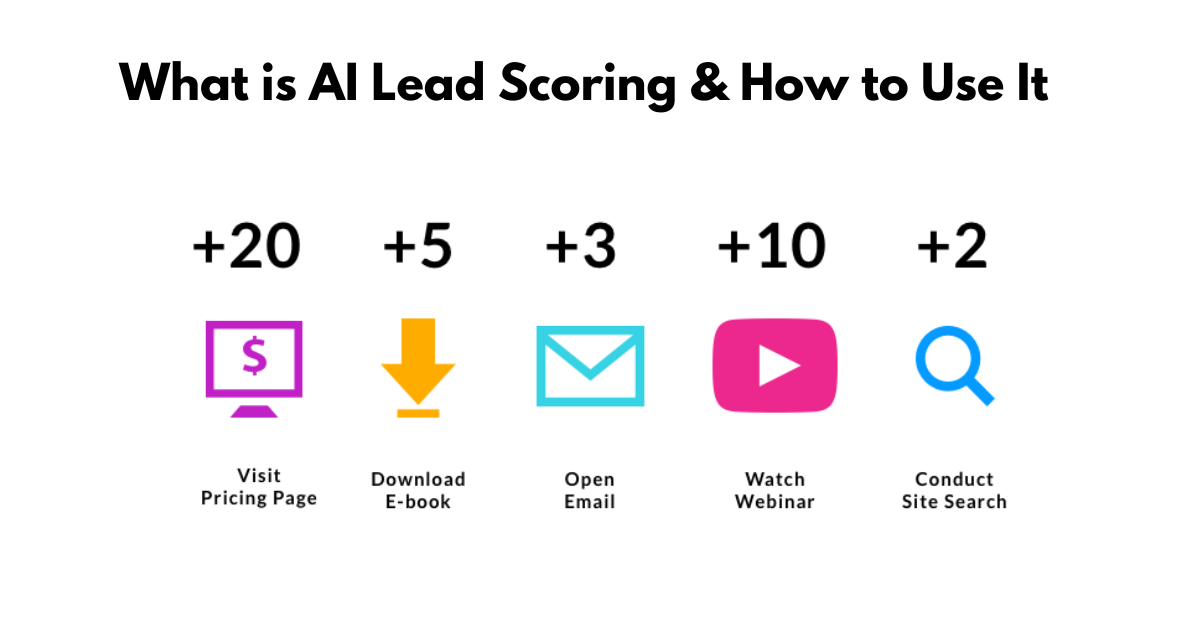
AI lead scoring is a method used by sales teams to rank prospects based on their likelihood to convert into customers. By 2024, AI technologies have refined this process, utilizing advanced algorithms to analyze historical data and behavioral signals. This enables businesses to focus their efforts on the most promising leads, thereby increasing the efficiency of their sales operations.
This blog will explain the fundamentals of AI based lead scoring and provide practical tips on how to effectively implement this technology to maximize your sales results in the coming year.
Why AI Lead Scoring?
AI lead scoring is a strategic approach that uses machine learning to evaluate the potential of leads, helping businesses prioritize their sales efforts more effectively. Traditional lead scoring often relies on static criteria and human judgment, which can be subjective and inconsistent. In contrast, AI lead scoring dynamically assesses lead data, including engagement metrics like website visits, email interactions, and social media activity, to calculate a score that predicts how likely a lead is to convert.
The use of AI for lead scoring allows for a more nuanced understanding of lead behavior. It can identify patterns and trends that are not immediately apparent, enabling sales teams to focus on leads with the highest conversion potential. This targeted approach not only improves efficiency but also increases the overall effectiveness of sales strategies, ultimately enhancing revenue generation.
What is Conversational AI Lead Scoring?
Conversational AI lead scoring is a process that uses artificial intelligence to evaluate and rank potential leads based on their interactions in conversational platforms, such as chatbots or virtual assistants. This technology analyzes the content of conversations, assessing factors like customer interest level, questions asked, and responses to prompts. By evaluating these interactions, conversational AI can determine the likelihood of a lead converting into a customer.
This approach allows businesses to prioritize their engagement efforts more effectively by focusing on leads that exhibit a higher potential for conversion. It streamlines the sales process, ensures a better allocation of resources, and enhances the overall customer experience by responding more accurately to individual needs and preferences. Conversational AI lead scoring is becoming an essential tool in the digital sales and marketing toolkit, particularly as businesses seek to optimize their online interactions.
Benefits of AI Lead Scoring
AI lead scoring offers several key benefits that can significantly enhance the effectiveness of sales and marketing efforts:
- Increased Efficiency: AI lead scoring automates the process of evaluating and ranking leads, saving valuable time for sales teams. This automation allows them to focus more on engaging high-potential leads rather than sifting through large volumes of data manually.
- Improved Accuracy: By leveraging historical data and machine learning, AI can more accurately predict which leads are most likely to convert. This reduces the guesswork involved in lead prioritization and increases the precision of targeting efforts.
- Enhanced Personalization: AI lead scoring can identify specific needs and interests of potential customers based on their interactions and behaviors. This insight enables sales and marketing teams to tailor their communications and offers, leading to more personalized customer experiences.
- Scalability: As businesses grow, managing leads manually becomes increasingly challenging. AI for sales lead scoring systems can easily scale to handle larger data sets and more complex lead qualifications, facilitating growth without compromising lead management quality.
- Better Resource Allocation: With a clearer understanding of which leads to prioritize, companies can allocate their resources more effectively, ensuring that marketing budgets and sales efforts are focused where they are likely to yield the highest returns.
- Faster Response Times: AI systems can score leads in real-time, enabling immediate engagement with potential customers at moments when they are most receptive. This quick response can significantly improve conversion rates.
How to Build an AI Lead Scoring Model?
Building an AI lead scoring model involves a structured approach to effectively rank and prioritize leads based on their likelihood to convert. Here’s how you can build an AI lead scoring model step-by-step:
- Data Collection:
Gather comprehensive data from various sources such as CRM systems, website interactions, social media activity, and email responses. This data should include demographic information, behavioral signals, and engagement metrics.
- Data Preparation:
Cleanse and preprocess the data to remove inaccuracies and inconsistencies. Normalize data formats and handle missing values to ensure the AI model has quality input for accurate predictions.
- Feature Selection:
Identify and select the most relevant features (variables) that influence a lead's likelihood to convert. This might include activity level, product interest, and communication responsiveness, among others.
- Model Development:
Choose an appropriate AI algorithm for lead scoring, such as logistic regression, decision trees, or neural networks. Train the model using historical data where the outcomes are known (e.g., whether the lead converted).
- Model Training and Validation:
Train the model on a subset of your data and validate its accuracy using another set (validation dataset). This process helps fine-tune the model to improve its predictive power and avoid overfitting.
- Integration:
Integrate the AI lead powered scoring model into your sales or CRM platform so it can automatically score new leads as they enter the system. Ensure it can update scores dynamically as new data becomes available.
- Monitoring and Updating:
Continuously monitor the model’s performance and make adjustments as necessary. Update the model periodically with new data and refine the features or algorithm to adapt to changes in lead behavior or market conditions.
- Feedback Loop:
Implement a feedback mechanism to capture the outcome of each lead (converted or not). Use this data to further refine and train your AI model, thereby enhancing its accuracy over time.
3 Best AI Lead Scoring Platforms
Here's a detailed look at three leading AI lead-scoring solutions, highlighting their features, pros, and cons:
HubSpot Sales Hub
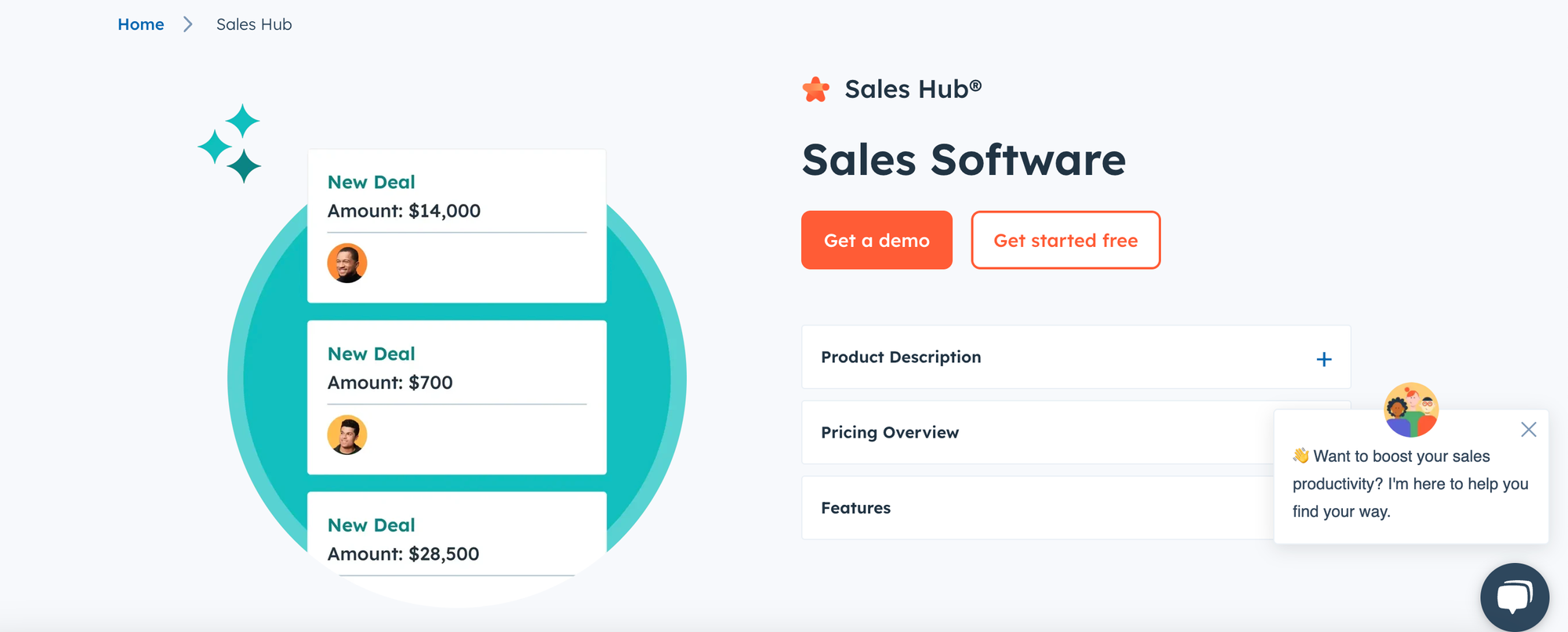
Features: HubSpot Sales Hub integrates AI and machine learning to automate lead scoring based on user-defined criteria and lead scoring analysis. It offers seamless integration with HubSpot's CRM and marketing tools, providing a unified view of lead activities.
Pros: The platform is user-friendly, offering intuitive navigation and integration with a wide range of marketing tools. Its real-time analytics help refine sales strategies effectively.
Cons: It can be expensive for smaller businesses, especially when scaling up the features. The system’s comprehensive nature may require a steeper learning curve for some users.
Salesforce Einstein
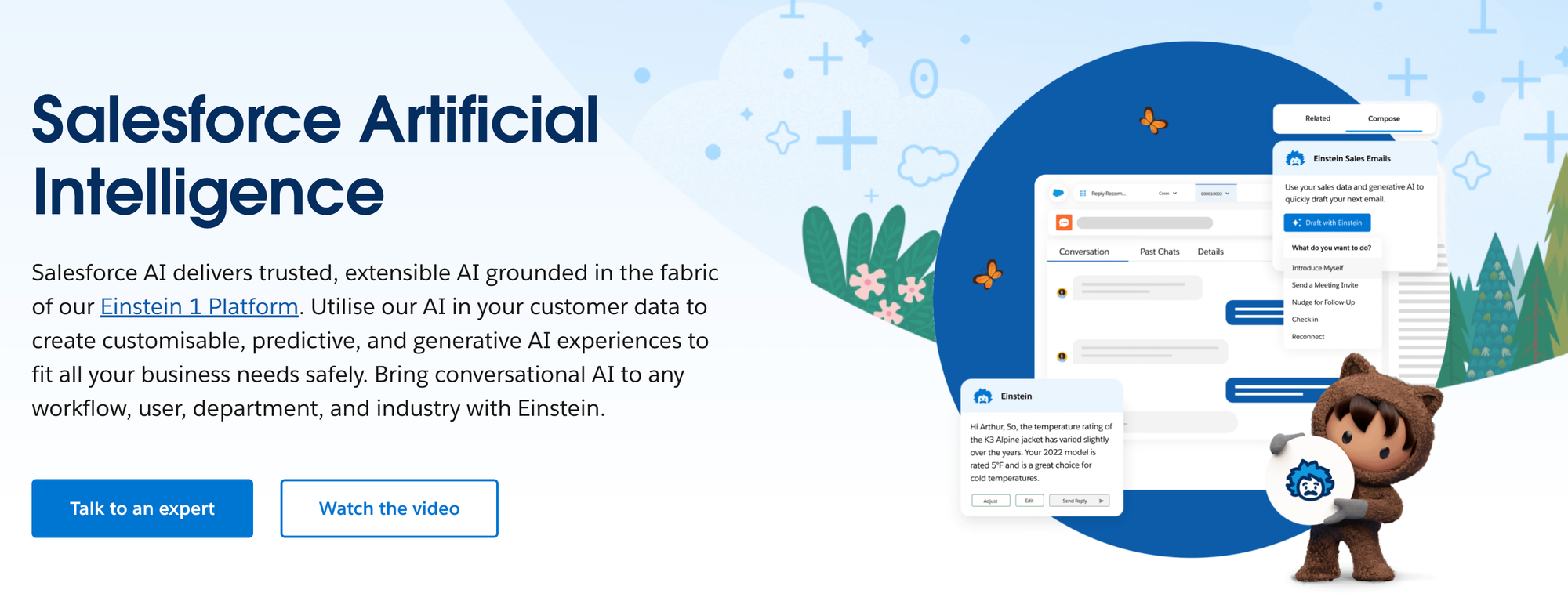
Features: Salesforce Einstein uses advanced AI to automatically score and prioritize leads based on past dealings and predictive insights. It's deeply integrated with Salesforce CRM, enhancing data synchronization across sales and marketing.
Pros: Highly customizable and scalable, suitable for businesses of all sizes. Offers powerful insights into sales forecasting and customer behavior.
Cons: Complexity and cost are significant, with customization requiring technical expertise. The platform might be too robust for smaller teams or those with simpler needs.
Pardot
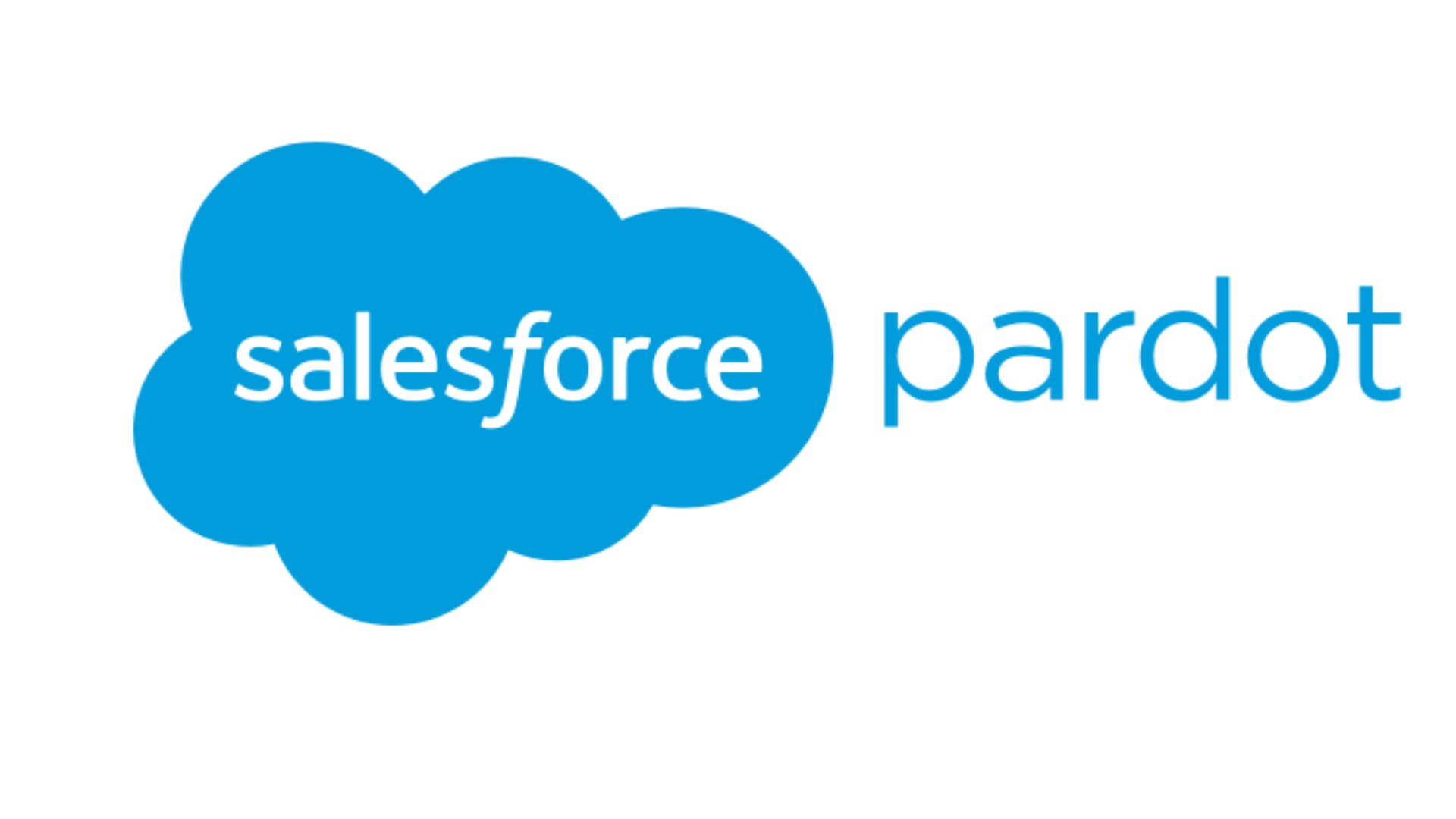
Features: Pardot, a Salesforce company, provides a marketing automation lead-scoring that includes an AI-powered lead scoring system. It scores leads based on their interaction with marketing content and demographic information.
Pros: Offers tight integration with Salesforce CRM, enhancing alignment between sales and marketing teams. It supports a wide range of automation features that streamline campaign management.
Cons: Pardot's pricing is on the higher side, making it less accessible for small to medium-sized enterprises. The interface and certain processes may also require a learning period to master effectively.
Conclusion
AI lead scoring represents a pivotal advancement in refining sales processes. By 2024, its application will enable businesses to prioritize their sales efforts more effectively, focusing on leads most likely to convert. This not only optimizes resource allocation but also enhances overall sales productivity and effectiveness. Implementing AI lead scoring requires careful consideration of data, features, and integration with existing systems, but the potential benefits in terms of increased conversions and streamlined operations make it a worthwhile investment for businesses aiming to stay competitive in the evolving marketplace.